Evaluation of the implementation of machine learning algorithm K-Nearest Neighbors (KNN) using rapid miner on junior high school student learning outcomes
DOI:
https://doi.org/10.31629/jg.v8i2.6590Keywords:
maximum K-Nearest Neighbors (KNN), rapidminer, classifier, learning outcomesAbstract
The focus of the PISA 2022 assessment is on the subjects of Mathematics, Language and Science. Therefore, these subjects are compulsory subjects at every level of education. Because learning activities are the most important activities, the success of a learning activity is measured by the learning outcomes that have reached completeness or failed. Prediction of completeness or failure can be done by classifying data using the K-Nearest Neighbors (KNN) algorithm using the RapidMiner application. The KNN algorithm is one of the classification methods for a set of data based on learning data that has been classified before. The data used are student learning outcomes in Mathematics, Indonesian Language and Science subjects at the junior high school education level in Padang city. This research aims to predict student learning outcomes in Mathematics, Indonesian and Science subjects based on student score completeness by comparing various k values to obtain the best performance of this algorithm. The results obtained after analyzing the KNN algorithm are Classification using the KNN algorithm is most accurate when the value of k = 5 and k = 7. Where by using the value of k, the accuracy of the KNN algorithm reaches the maximum result of 94.12%. Thus, this algorithm can help teachers to predict or find out how appropriate student completeness.
Downloads
References
Cholil, S.R., Handayani, T., Prathivi, R., Ardianita, T. (2021). Implementasi algoritma klasifikasi k-nearest neighbor (KNN) untuk klasifikasi seleksi penerima beasiswa. IJCIT (Indonesian Journal on Computer and Information Technology) Vol. 6 No.2, November 2021 Hal. 118-127.
Fajri, M. S., Septian, N., & Sanjaya, E. (2020). Evaluasi implementasi algoritma machine learning k-nearest neighbors (KNN) pada data spektroskopi gamma resolusi rendah. Al-Fiziya: Journal of Materials Science, Geophysics, Instrumentation and Theoretical Physics, 3(1), 9–14. https://doi.org/10.15408/fiziya.v3i1.16180
Farokhah, L. (2020). Implementasi k-nearest neighbor untuk klasifikasi bunga dengan ekstraksi fitur warna RGB. Jurnal Teknologi Informasi Dan Ilmu Komputer, 7(6),1129–1136. https://doi.org/10.25126/jtiik.2020722608
Jabbar, M. A., Deekshatulu, B. L., & Chandra, P. (2013). Classification of heart disease using k- nearest neighbor and genetic Algorithm. Procedia Technology, 10, 85–94. https://doi.org/10.1016/j.protcy.2013.12.340
OECD, T. O. for E. C. and D. (2023). Program for international student (PISA) 2022 assessment and analytical framework. https://www.oecdlibrary.org/education/pisa-2022-assessment-and-analytical framework_dfe0bf9c-en
Rahim, U., & Rafiun, A. (2012). Peran matematika dalam menunjang mata pelajaran IPA, bahasa inggeris dan bahasa indonesia. Jurnal Pendidikan Matematika, 3. https://www.neliti.com/publications/317669/peran-matematika-dalam-menunjang-mata-pelajaran-ipa-bahasa-inggeris-dan-bahasa-i
Panjaitan., CHP., Pangaribuan., L.J., Cahyad., C.I. (2022). Analisis metode k-nearest neighbor menggunakan rapid miner untuk sistem rekomendasi tempat wisata labuan bajo. Remik: Riset dan E-Jurnal Manajemen Informatika Komputer, Volume 6, Nomor 3, Agustus 2022 http://doi.org/10.33395/remik.v6i3.11701
Sichao, Z., (2020). Cost-sensitive KNN classification. Neurocomputing, vol. 391, pp. 234–242
Sumarlin, S. (2016). Implementasi algoritma knearest neighbor sebagai pendukung keputusan klasifikasi penerima beasiswa PPA dan BBM. Jurnal Sistem Informasi Bisnis, 5(1), 52–62. https://doi.org/10.21456/vol5iss1pp52-62
Solchan, H. 1998. Interaksi belajar mengajar bahasa indonesia. (Jakarta: Depdikbud)
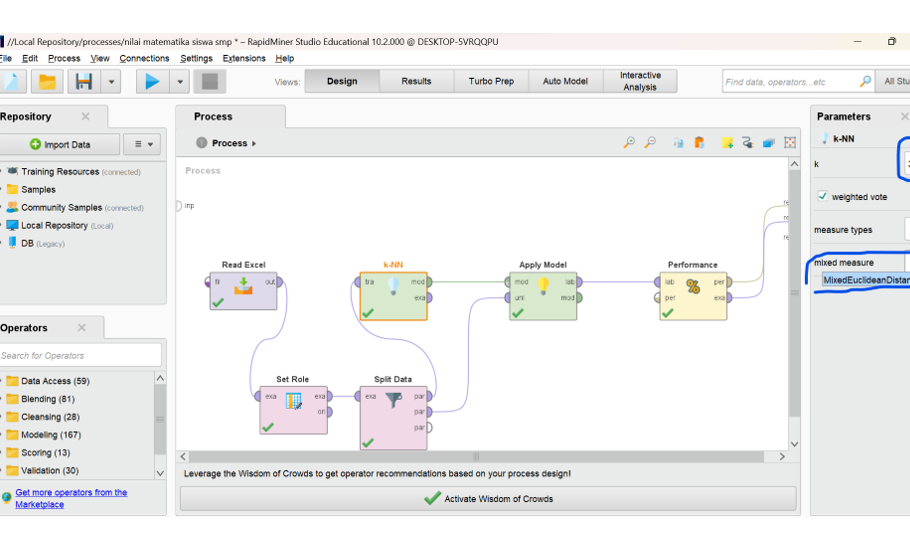
Downloads
Published
How to Cite
Issue
Section
License
Copyright (c) 2023 Jurnal Gantang

This work is licensed under a Creative Commons Attribution-NonCommercial-ShareAlike 4.0 International License.